Moshe holds B.Sc (Software Engineering), M.Sc (Software and Information Systems Engineering), and PhD. (Software and Information Systems Engineering) from Ben-Gurion University (BGU) of the Negev, Israel. His research work focuses on Personalization, Data Mining, Machine Learning and Recommender Systems with emphasis to context inference with deep learning techniques and its usage in recommender systems. He is currently a lecturer at the Coller School of Management, Tel Aviv University. He has recently finished a postdoctoral researcher position at NYU Stern School of Business and an associate research scientist at the NYU Stern Fubon Center of Technology, Business and Innovation.
Dr. Moshe Unger
Coller School of Management
הפקולטה לניהול ע"ש קולר
סגל אקדמי בכיר
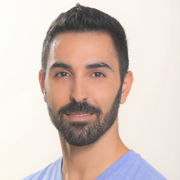
General Information
CV
Fields of Research
Recommendation systems, big data, machine learning, deep learning, cyber security.
Selected Publications
- Bauman, Konstantin, Alexander Tuzhilin, and Moshe Unger. "HyperCARS: Using Hyperbolic Embeddings for Generating Hierarchical Contextual Situations in Context-Aware Recommender Systems." Information Systems Research (2024).
- Unger, M., Wedel, M. & Tuzhilin, A. Predicting consumer choice from raw eye-movement data using the RETINA deep learning architecture. Data Min Knowl Disc (2023).
- Unger, Moshe, et al. "Don’t Need All Eggs in One Basket: Reconstructing Composite Embeddings of Customers from Individual-Domain Embeddings." ACM Transactions on Management Information Systems (2023).
- Unger, M., Tuzhilin, A., & Livne, A. (2020). Context-Aware Recommendations Based on Deep Learning Frameworks. ACM Transactions on Management Information Systems (TMIS), 11(2), 1-15.
- Unger, M., & Tuzhilin, A. (2020). Hierarchical Latent Context Representation for Context-Aware Recommendations. IEEE Transactions on Knowledge and Data Engineering (TKDE).
- Unger, M., Shapira, B., Rokach, L., & Livne, A. (2018). Inferring contextual preferences using deep encoder-decoder learners. New Review of Hypermedia and Multimedia, 24(3), 262-290.
- Unger, M., Bar, A., Shapira, B., & Rokach, L. (2016). Towards latent context-aware recommendation systems. Knowledge-Based Systems, 104, 165-178.
Publications in Refereed Conferences
- Unger, M., Shapira, B., Rokach, L., & Bar, A. (2017). "Inferring contextual preferences using deep auto-encoding", In Proceedings of the 25th Conference on User Modeling, Adaptation and Personalization (pp. 221-229). ACM.
- Bar, A., Shapira, B., Rokach, L., & Unger, M. (2016). "Scalable attack propagation model and algorithms for honeypot systems", In Big Data (Big Data), 2016 IEEE International Conference on (pp. 1130-1135). IEEE.
- Bar, A., Shapira, B., Rokach, L., & Unger, M. (2016). "Identifying Attack Propagation Patterns in Honeypots Using Markov Chains Modeling and Complex Networks Analysis", In Software Science, Technology and Engineering (SWSTE), 2016 IEEE International Conference on (pp. 28-36). IEEE.
- Unger, M., Shapira, B., Rokach, L., & Bar, A. (2016). "Deep Auto-Encoding for Context-Aware Inference of Preferred Items' Categories", In RecSys Posters.
- Unger, M. (2015). "Latent context-aware recommender systems", In Proceedings of the 9th ACM Conference on Recommender Systems (pp. 383-386). ACM.
- Unger, M., Bar, A., Shapira, B., Rokach, L., & Gudes, E. (2014). "Contexto: lessons learned from mobile context inference", In Proceedings of the 2014 ACM International Joint Conference on Pervasive and Ubiquitous Computing: Adjunct Publication (pp. 175-178). ACM.